Call for posters: SimTech2023
Verlängerte Frist für die Einreichung von Posterabstracts: 7. April 2023
Der weitere Inhalt des Beitrags ist nur auf Englisch verfügbar.
Call for Posters for SimTech2023
The University of Stuttgart and the Cluster of Excellence EXC 20275 "Data-integrated Simulation Science" (SimTech) extended the deadline for poster abstract submission for SimTech2023 to April 7, 2023.
They invite authors to submit short abstracts for poster presentations of new ideas, work in progress and promising new results in all areas of data-integrated simulation – from (hybrid) models for biological, environmental or technical systems and material over numerical methods for partial differential equations and learning, stochastic (Bayes) approaches for uncertainty quantification, reduction and optimization, dynamical systems and control to high performance computing for hybrid and novel architectures including quantum computing.
One or more authors of accepted posters are expected to present the poster in person at the conference. Accepted posters and the corresponding short abstracts will be published online via the conference website.
Please submit your abstract as a single pdf file to conference_ps@simtech.uni-stuttgart.de using the latex template attached here.
Fell free to forward this call to interested colleagues! We are looking forward to welcoming you in Stuttgart.
Timeline
- Deadline for poster abstract submission: April 7
- Notification of acceptance: April 30
- Early Registration Deadline: May 15.
The SimTech2023 Conference
Most parts of the presentation program including keynote speakers are published at the conference webpage:
The conference will cover topics such as
- classical computational science enhanced by learning based components,
- data-based scale bridging,
- high performance computing,
- numerical solvers optimized by meta-data based methods,
- machine learning for simulation and visualization,
- data-based (surrogate) modeling for interactive applications and control problems,
- Bayesian approaches for uncertainty reduction and model assessment and many more.
Zugehörige Artikel
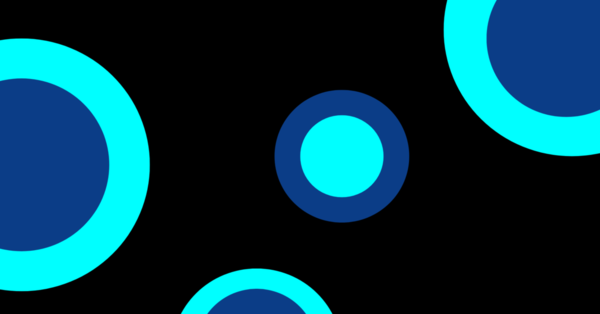
IMPRS-IS Call for Applications is Now Open

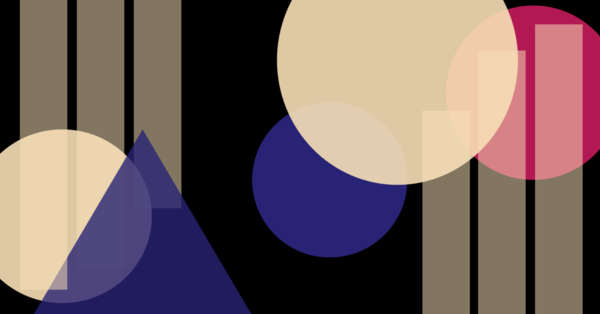
Extended deadline for Cyber Valley Entrepreneurial Programs

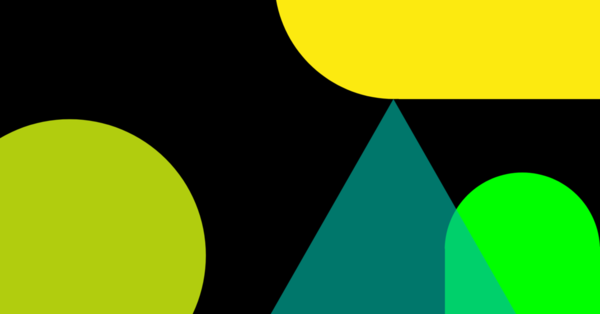
Universität Stuttgart mit Clusterskizzen erfolgreich
