Knowledge-driven machine learning and its applications
International SimTech Summer School
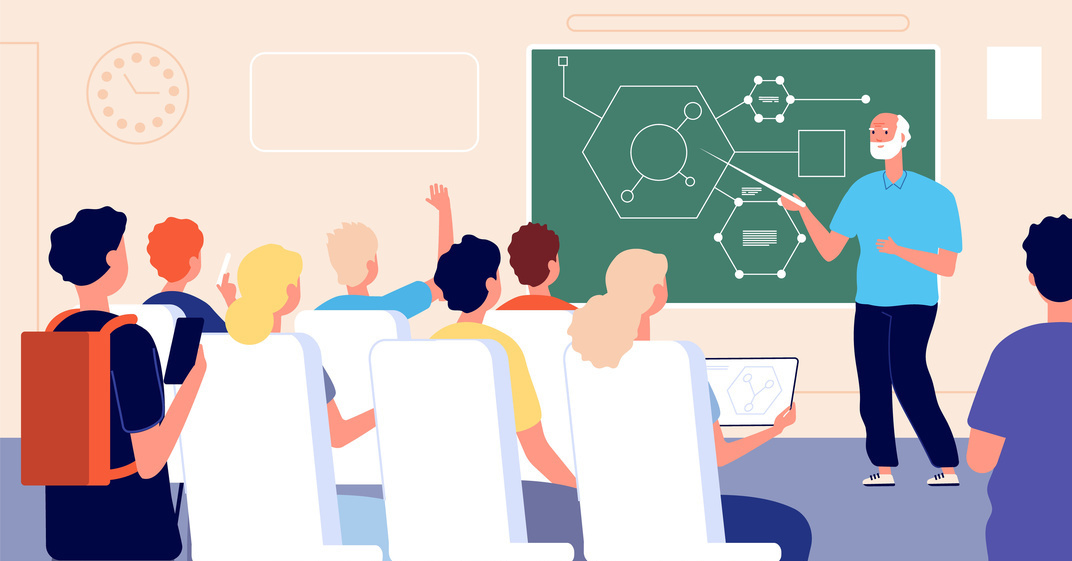
Despite the remarkable progress in machine learning (ML) techniques and, more generally, data-driven artificial intelligence frameworks in the last decades, most ML approaches are currently unable to extract interpretable information and knowledge from data. Moreover, predictions obtained from purely data-driven models may be physically inconsistent or even implausible. It is currently highly investigated how to include existing (expert) knowledge into the ML design to counteract these challenges. The careful integration of prior knowledge about a process, such as physical or chemical insights, into the learning procedure not only improves the quality of the learned representation but also tends to speed up the learning process with fewer data samples.
This summer school aims to equip the participants with a basic understanding of how different aspects of knowledge, including but not limited to established simulation paradigms, can be induced in modern learning architectures.
Confirmed speakers
- Prof. George Em Karniadakis and Prof. Khemraj Shukla (both Brown University)
- Prof. Tilman Plehn and Dr. Anja Butter (both University of Heidelberg)
- Prof. Pietro Faccioli (University of Trento) and
- Prof. Pascal Friederich (Karlsruhe Institute of Technology)
Audience
The SimTech Summer School is primarily intended for Master's students, PhD reseachers, and early-career PostDocs.