International team of scientists develops machine learning model that could help inform COVID-19 containment measures
Newly published paper presents research that could make an important contribution to discussions about policies to ease lock-down measures
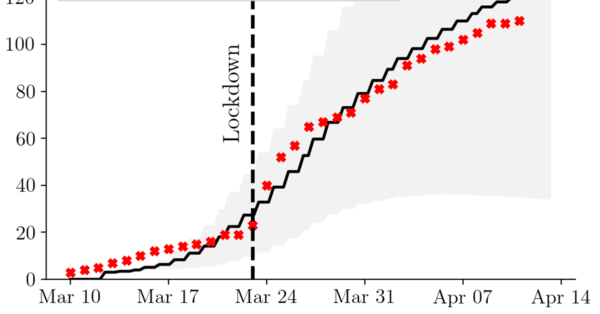
Tübingen – Scientists from the Max Planck Institutes for Intelligent Systems and Software Systems, ETH Zurich, the École Polytechnique Fédérale de Lausanne, and the global public health non-profit Zerobase Foundation, have developed a new mathematical model that could help inform COVID-19 containment measures by drawing on data from contact tracing technologies. The authors Lars Lorch, William Trouleau, Stratis Tsirtsis, Aron Szanto, Bernhard Schölkopf, and Manuel Gomez-Rodriguez presented their findings in a paper entitled “A Spatiotemporal Epidemic Model to Quantify the Effects of Contact Tracing, Testing, and Containment,” which was released last week. The scientists discussed the research project at last Wednesday's virtual “ELLIS against COVID-19” workshop.
"By using data from any of the contact tracing technologies currently available, the model we’ve developed can quantify or predict the potential effect - in terms of containment - that contact tracing, social distancing measures, or business restrictions will have on a specific town or city", says Manuel Gomez Rodriguez, faculty member at the Max Planck Institute for Software Systems in Kaiserslautern, Germany. The team recently put the new model to the test with a simulation on the town of Tübingen in southwestern Germany. Drawing on COVID-19 and mobility pattern data collected between March 10 and April 12, the model predicted that coronavirus infections would have skyrocketed to 29,000 (about a third of the local population) if social distancing measures had not been put in place. “If the model considers the restrictions that were implemented, it closely predicts the number of infections that actually occurred,” says Gomez-Rodriguez.
“What sets this research apart from other approaches is that it can specifically quantify the effects of tracing, testing, and containment strategies on individual people,” says Bernhard Schölkopf, Director at the Max Planck Institute for Intelligent Systems in Tübingen. “As policymakers around the world discuss strategies to gradually transition out of lock-down and reopen their economies, this model could help pave a safe way forward.” The paper also demonstrates that contact tracing technology such as PEPP-PT or Zerobase can also help flatten the curve. The authors conclude that an anonymous contact tracing system can enhance the effectiveness of conventional public health measures, thus helping contain and ultimately stop the spread of COVID-19.
Related Articles
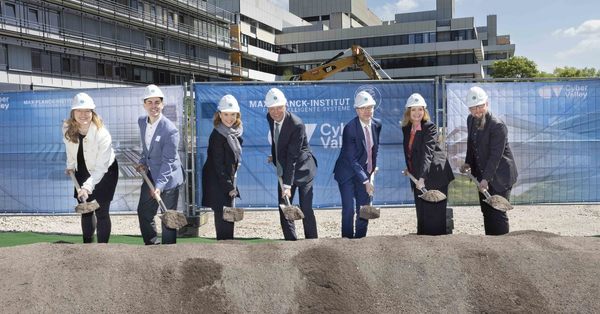
Groundbreaking Ceremony for the Cyber Valley Building in ...

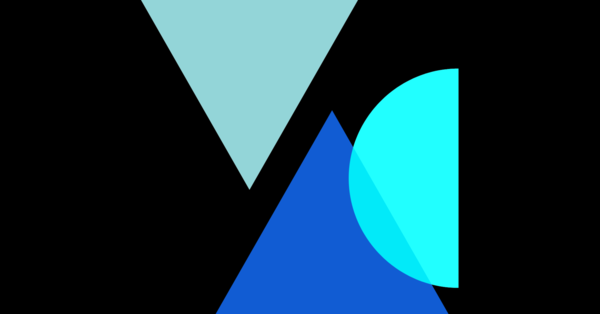
75 doctoral students join the Cyber Valley Innovation Campus

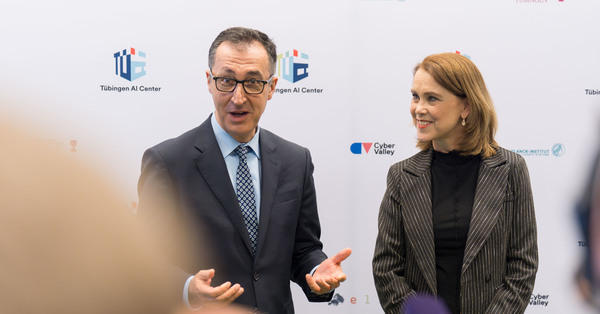
Cem Özdemir visits Cyber Valley
