“We’d like to make complex dynamical systems better able to adapt to changing conditions”
Michael Mühlebach recently joined MPI-IS as leader of the independent Learning and Dynamical Systems research group

Tübingen - Michael Mühlebach developed an interest in machine learning early on in his career. Over the course of his PhD, he designed learning and control algorithms for a balancing robot and a flying machine. While such research projects were based on hand-crafted solutions for the reliable operation of small-scale systems in a confined environment, Mühlebach is now looking to expand his research to the next frontier: as leader of the independent Learning and Dynamical Systems research group at the Max Planck Institute for Intelligent Systems (MPI-IS) in Tübingen, Mühlebach plans to focus his attention on large-scale dynamical systems that interact with complex environments. He joined MPI-IS on February 1, 2021.
“There is a lot of exciting research to be done at the intersection between machine learning, optimization, and control theory.” Mühlebach says. “We would like to make systems such as power grids or autonomous transportation systems better at adapting and responding to changing conditions and circumstances. Here, the challenge lies in developing algorithms that not only detect patterns in the data to continuously improve performance, but that are also capable of functioning reliably when something unexpected happens, like an extreme weather event or a sudden change in traffic, for example.”
At present, machine learning and control methods are widely applied to dynamical systems that have a few degrees of freedom and that work in a confined environment. For example, these methods are very effective at stabilizing an autonomous flying vehicle and ensuring it can adapt to crosswinds or the wear-and-tear of its propellers. However, hand-crafted solutions are still needed to reliably handle major and sudden changes, such as the loss of a propeller or a sudden and large change in payload. Another significant challenge lies in dealing with larger systems. To make machine learning algorithms more capable of succeeding in more complex scenarios, Mühlebach would like to pursue the approach of better incorporating prior knowledge in the form of physical laws, for instance. “Ultimately, the idea is to eliminate misleading patterns in the data, because these have a negative effect on a system’s ability to make accurate predictions. And even if something unexpected happens that the data doesn’t capture, the system still has to be able to cope, adapt, and function nonetheless. With systems that can affect people’s lives, the stakes are very high and reliability is crucial,” Mühlebach says.
Another central challenge in this field of research lies in developing approaches that can effectively handle the sheer amount of data, while still operating in real time. “As our sensing capabilities increase, we are able to collect more and more data and create very precise models. However, we would like to update our models and use them to take sensible action in real time. It is therefore decisive that our algorithms do their jobs in a short amount of time and effectively use the available computational resources.” For this reason, Mühlebach is expanding on his past research in the area of optimization algorithms. These are computer programs that can decide, for example, which action is favorable or even the best among a set of alternatives. “Ultimately, most machine learning, statistical inference, and control algorithms are based on mathematical optimization.”
As he builds his team, Mühlebach looks forward to taking advantage of the benefits that the MPI-IS and the Tübingen research community offer. “Tübingen is one of the leading places for machine learning, and I chose MPI-IS because there are excellent people here doing both theoretical and experimental work. The infrastructure is amazing; there aren’t many places like this in the world,” he says.
Mühlebach’s research is supported by the prestigious Branco Weiss and Emmy Noether Fellowships, which he received in 2018 and 2020, respectively. The Branco Weiss Fellowship supports outstanding and interdisciplinary research projects that depart from mainstream research. The Emmy Noether Fellowsip, a programme of the German Research Foundation DFG, provides outstanding early career researchers funding for a period of six years to build independent research groups. Previously, Mühlebach was a postdoctoral researcher at the University of California, Berkeley and completed his master’s degree and PhD at ETH Zurich.
Related Articles
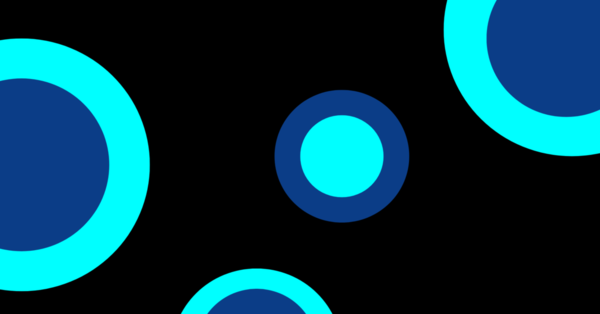
IMPRS-IS Call for Applications is Now Open

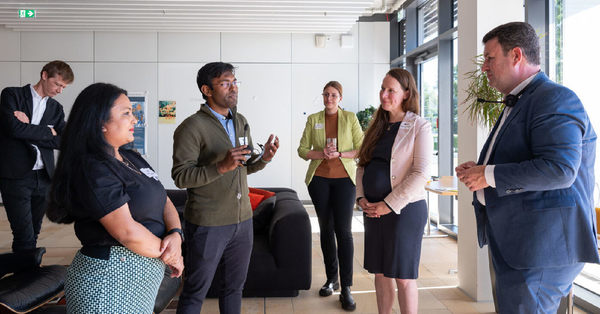
Federal Minister Hubertus Heil visits Cyber Valley in Tüb...

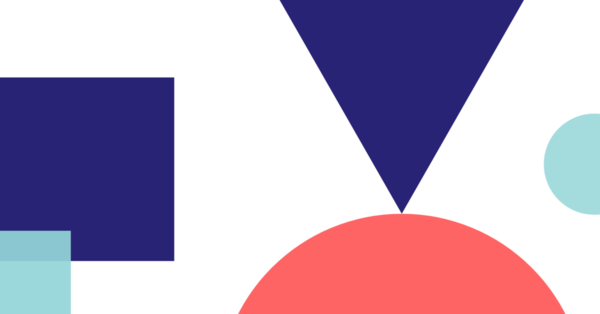
European Robotics Forum 2025 (ERF2025)
